A tool rationalization head start with BigPanda
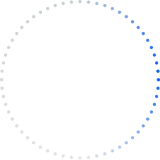
Tool rationalization, sometimes called tool consolidation, is the systematic analysis of observability and monitoring tools, the consideration of onboarding new tools to fill gaps, and the retirement of unnecessary tools. Perhaps you and your IT team are struggling with constantly buying new tools to meet a very niche use case to unlock new capabilities. Or maybe you’re looking to consolidate your observability tool stack but face challenges around monitoring noise, siloed data, and overlapping functionalities. If so, we will explain how BigPanda can help during your tool consolidation project by addressing those hurdles and provide an example of a BigPanda customer who was able to consolidate their tools and reduce costs.
The proliferation of observability and monitoring tools
This proliferation of observability and monitoring tools is also known as tool sprawl. The average organization using BigPanda is coordinating data from ~20 different observability solutions, and they report managing their observability sprawl is a constant challenge due to noise hiding vital, actionable alerts and siloed data workflows.
Tool rationalization takes time and coordination across the organization as there are different teams with unique specialties, and that means a lot of tools that can solve similar purposes or optimized for specific tasks. When discussing tool consolidation, IT leaders are in a constant tug-of-war trying to balance whether to consolidate tools to reduce costs and streamline processes or invest in more tools to push toward innovation. As a result, the topic of tool rationalization presents IT leaders with a fine line to walk.
While BigPanda is not a tool rationalization vendor, nor is there one single magic bullet to stop tool sprawl, BigPanda helps IT operations deliver an unbiased, starting view of each observability tool’s impact on the incident management process.
Note: In this blog, we are referring to a hypothetical custom-built observability tool called OEM to avoid indicating the value/quality of a specific vendor offering. It is not an actual tool but simply used for reference.
How can BigPanda help with tool rationalization?
BigPanda can help you with your observability tool rationalization by:
- Identifying the volume and quality of alert data across teams and tools
- Understanding how NOC or SRE teams act on observability alerts and identifying opportunities to increase the value of existing observability data using enrichment.
- Gaining additional insights into the downstream impact observability has on incident response and where gaps or redundancies may lay
- Pointing you in the right direction to improve tools and measure these changes
“BigPanda’s Unified Analytics helps unify monitoring and observability data into a single pane of glass. The dashboards helps us avoid surprises and illuminate ways we can optimize incident management workflows.” —Lukas Johnson, Lead IT Infrastructure Engineer, Lumen Technologies
Get the full picture of your alert quality
Before you think about consolidation, gaining visibility into what is happening across your entire organization is difficult. What’s harder is determining how much value they’re bringing to your organization and whether it’s enough value to keep them in your stack.
BigPanda seamlessly combines fragmented data from various sources across your organization with the alarms generated by your observability tools. In essence, BigPanda ingests data from your observability tools, refines it through deduplication, correlation, normalization, and enrichment, and presents a unified, unbiased view of events across your entire IT operation in the BigPanda Unified Analytics platform.
As part of Unified Analytics, there are out-of-the-box dashboards to evaluate your observability tools objectively. For example, the Monitoring Sources Dashboard provides a big picture of all the alerts and their quality being ingested into BigPanda from your observability stack. In the image above, you can see that this stack is ingesting over 120,000 alerts from 10 different tools, with many being classified as low, medium, and high-quality alerts. BigPanda classifies alerts by implementing specific criteria to assess their contribution to actionability.
Understanding the value of alerts
Once you’ve gained an overarching perspective of your observability toolset, you can leverage BigPanda to assess the effectiveness of each tool.
From your initial investigation in the Monitoring Sources Dashboard, you may have noticed in the Sankey diagram that OEM only produces 4% of alerts out of all the observability tools in the stack. But, most of the alerts coming from OEM are low-quality alerts that either are ignored by NOC teams or never addressed despite tickets being opened.
Implications downstream
After establishing that OEM produces low-quality alerts, it would be essential to understand how these low-quality alerts affect your IT environment and teams downstream. To understand this, we can utilize the ITSM Tools Dashboard.
For OEM specifically, you can see that a majority of the tickets are considered P4. Immediately, this indicates that the tickets created for OEM are low priority and take up the responder’s time when they could focus on P2 tickets or higher. You can dive deeper using the MTTx Breakdown Dashboard to see how tickets created from OEM events slow down your team from responding to other incidents.
Addressing gaps
Continuing your investigation into OEM, you may ask, “Why is it generating low-quality alerts, and how is no one acting on them?” By utilizing the newly released Alert Quality Dashboard, you can identify that OEM generates low-quality alerts due to the absence of established runbooks, impact, owners, and enrichment data. This absence makes it challenging to discern impacted areas and other dependencies, rendering these alerts non-actionable without the necessary context.
You can also look at the Tag Standardization Dashboard to understand how OEM alerts are tagged. From this visualization, you can see that OEM alerts are missing defined tags, which can contribute to low-quality alerts and low actionability.
Iterate and collaborate: Charting the course forward
After identifying that OEM alerts are low quality, you can work with the OEM tool owner to implement changes to increase alert quality and actionability. For example, you can work on standardizing alert tags that fit in with the greater observability stack. You can also work on implementing a runbook to automate ticket creation and free up time for your NOC team. These changes you make can be measured utilizing the same dashboards used to identify these gaps initially. For example, the Alert Quality Dashboard, can evaluate if more alerts are classified as higher quality and, more importantly, see if they are actionable by NOC teams.
If OEM continues to produce low-quality, unactionable alerts, this might be a signal to consider consolidation because it produces little value and could distract operators from more critical alerts. If you notice substantial improvements, such as increased high-quality, actionable alerts, you can explore other tools utilizing the same dashboards you used to understand OEM’s utility.
IHG gains visibility to reduce costs and increase actionable alerts
IHG used BigPanda to gain an overview of their observability stack. IHG owns, leases, and manages over 6,000 hotels worldwide, with 1,800 more properties in development. As IHG expanded, the Unified Command Center (UCC), IHG’s centralized monitoring and alerting organization, experienced an overwhelming influx of events from all their observability tools that made it difficult to identify actionable alerts from event noise, causing incidents and outages to occur.
IHG utilized BigPanda to gain a centralized overview of its observability environment. This enabled the UCC to filter out false positive alerts, identify and consolidate redundancies, and add context to events from underperforming observability tools to transform them into actionable alerts. Overall, IHG increased the availability of key applications while reducing costs and IT complexity.
Begin your tool rationalization journey with BigPanda
We understand tool consolidation is a long process. BigPanda aims to alleviate the challenges associated with evaluating your observability tools. We provide you with essential quantitative context that allows you to establish a baseline understanding which empowers you to make more informed decisions to improve the effectiveness of the tools in your environment.
To learn more about how BigPanda can help you in your rationalization process, get your own personalized demo.