The BigPanda Event Enrichment Engine
BigPanda provides cross-domain alert enrichment with rich topological context so operators can identify meaningful patterns and quickly take action to prioritize and mitigate major incidents.
Future-proof AIOps with seamless enrichment of IT data
Ingest, normalize, filter, and enrich data to deliver context-rich alerts and help teams identify meaningful patterns to rapidly identify, investigate, and resolve incidents.
Data extraction
Ingest and extract values hidden in the alert and/or contained in contextual data, and add them as enriched data to the alert.
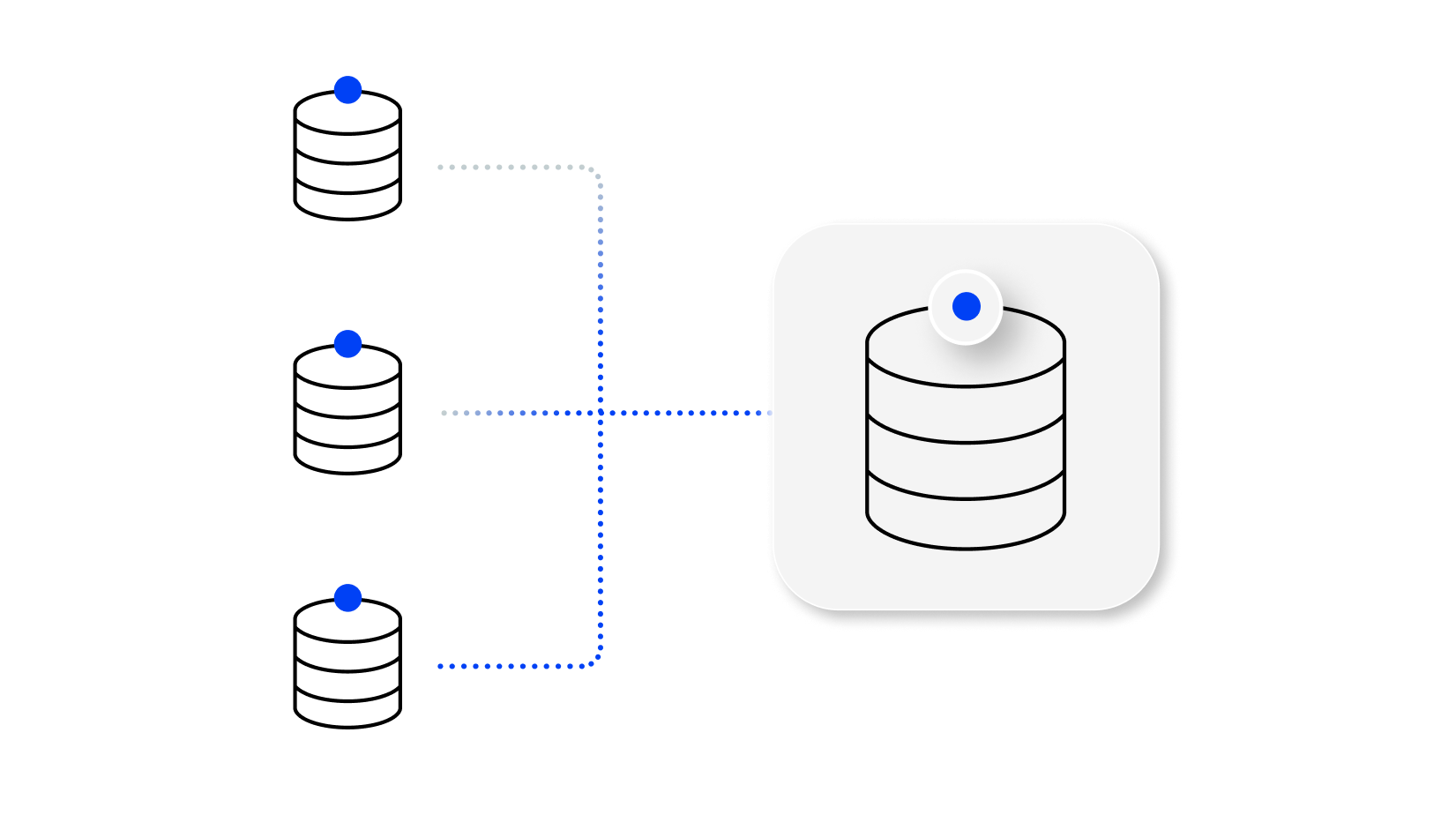
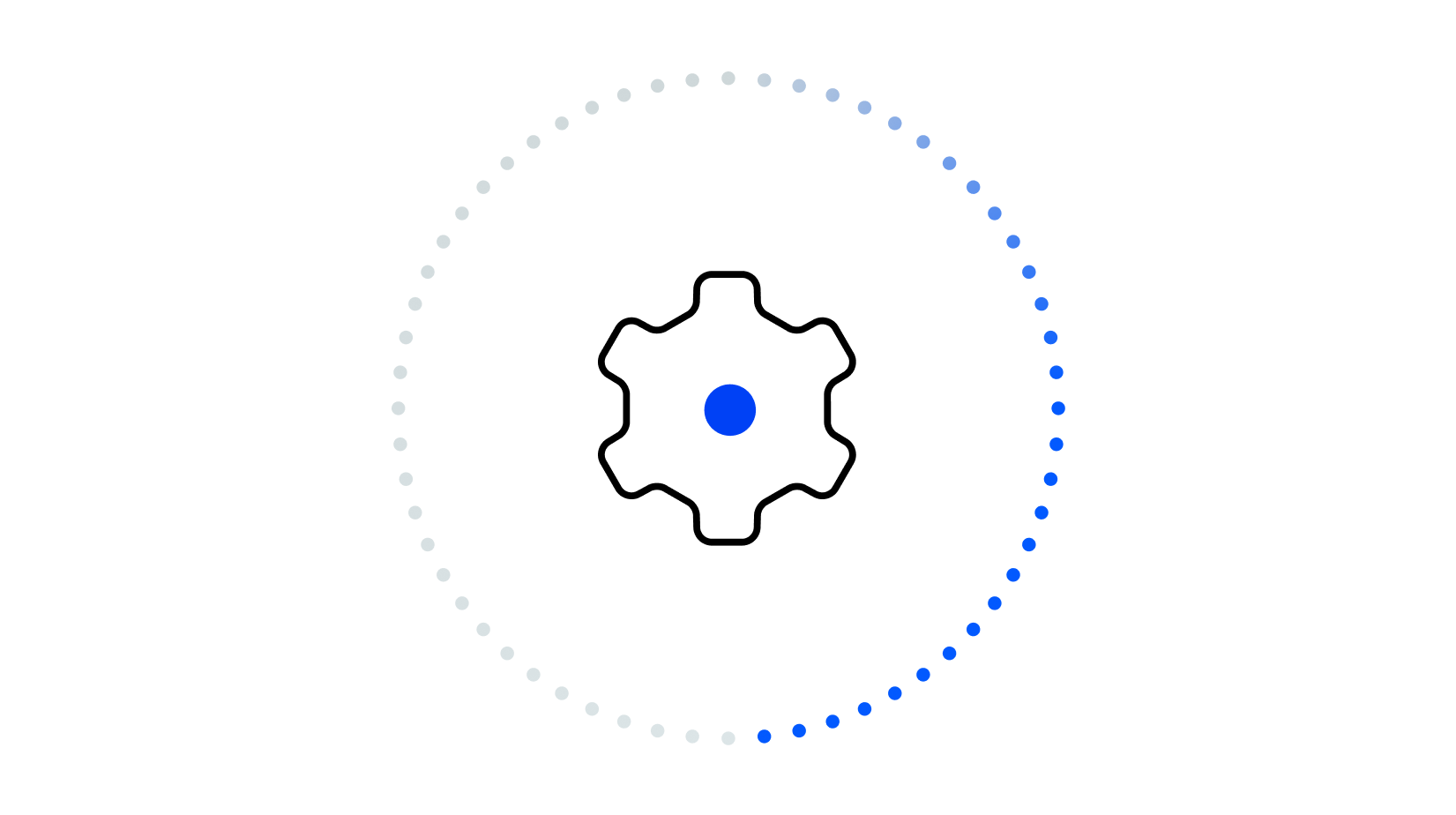
Data composition
The Event Enrichment Engine combines several alert and contextual data values from across silos and adds them to the alert as a new enrichment tag.
Mapping enrichment
Ingest relational data from discovery & mapping tools, to identify impact across IT environments.
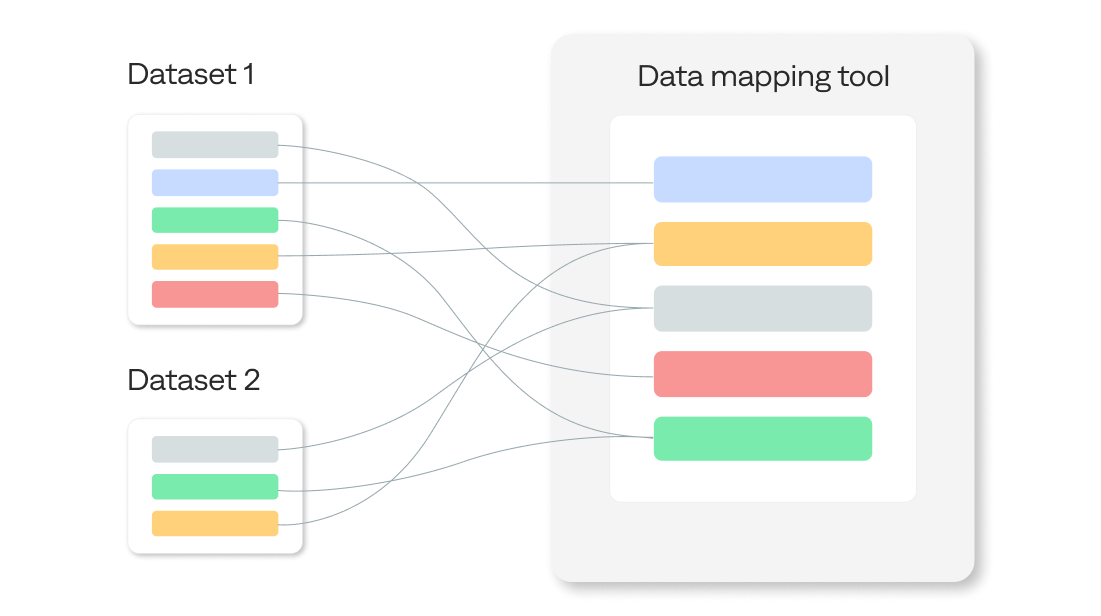
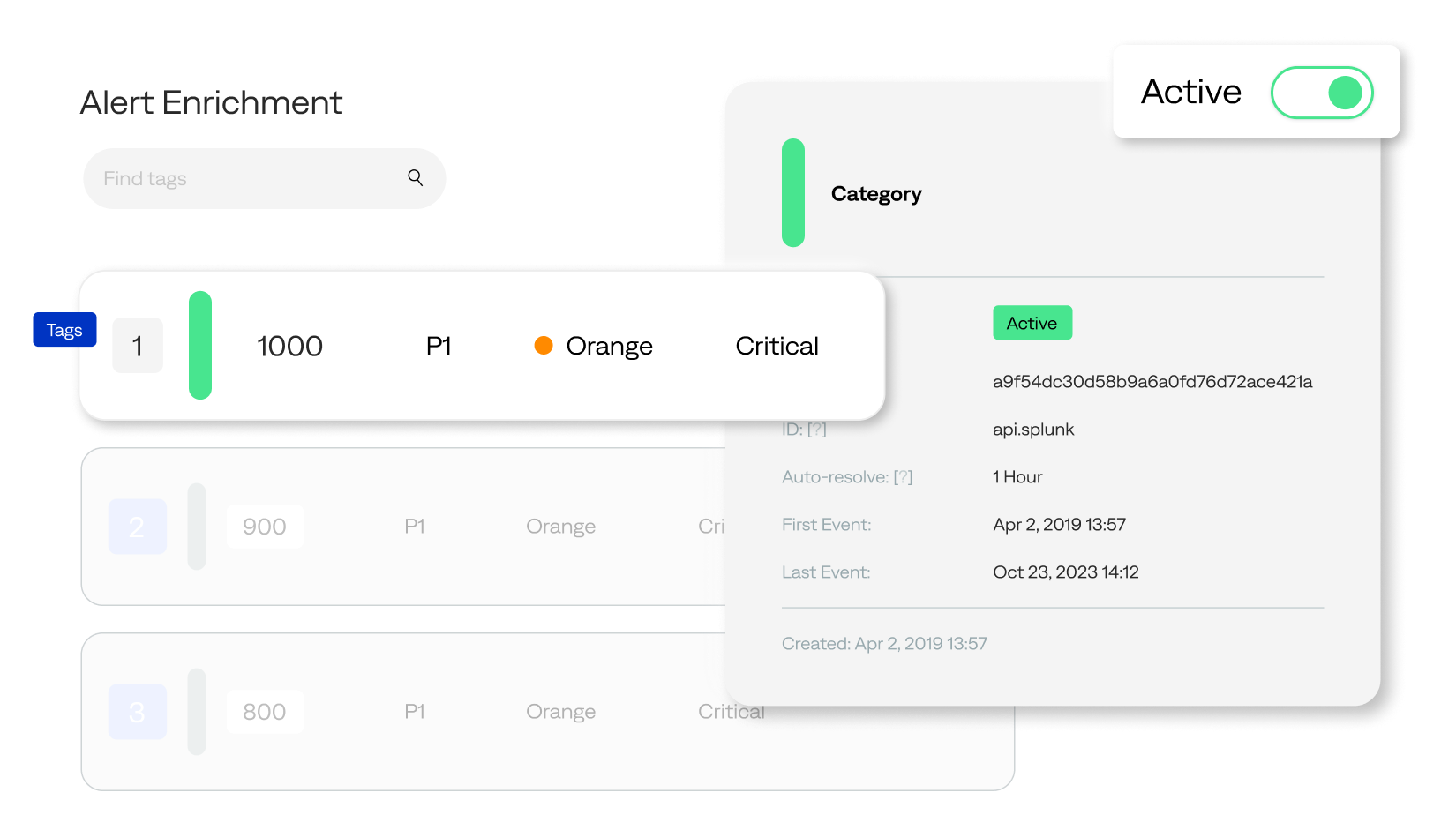
Enriched alerts for actionable AIOps
Enrich alerts with all the necessary context and data to reduce noise, enhance probable root cause determination, and improve workflow automations.
Improved correlation and alert noise suppression
“For us, an alert is not actionable unless it comes into BigPanda, is enriched, and is potentially correlated with the other alerts in the system.”
More accurate root cause analysis
“BigPanda helps us detect incidents and uncover probable root cause in real-time, which significantly reduces our MTTR.”
Enhanced automatic workflows
“BigPanda has helped significantly with deduplicating, correlating, and automating our process. The enrichment data we process through BigPanda enables us to create more specific and insightful alert tags.”
FAQ
How does the Event Enrichment Engine enrich data for improved context on alerts and incidents?
BigPanda uses topology, CMDB, change, and historical-based alert correlation to correlate alerts on various dimensions, such as application, physical topology, cluster, check, tags, etc. This enables operators to identify relational patterns across their IT infrastructure, anticipate issues, and get ahead of issues before they cause major outages.
Does the Event Enrichment Engine use correlation?
The BigPanda Event Enrichment Engine normalizes, enriches, and correlates data across services and applications, and on various attributes. BigPanda filters disparate alerts across multiple observability tools into digestible incidents in a single pane of glass to streamline incident understanding and resolution. Correlation patterns are also tunable to your specific organizational needs.
How is enrichment from BigPanda different from other AIOps vendors?
BigPanda ingests data from multiple data sources (on average 20), to include observability, change, topology, and CMDB data. BigPanda can also stream additional sources of data beyond MELT to give context about change, historical, topological, inventory, and more. By uniting infrastructure-wide data, BigPanda allows operators, SREs, and DevOps to see meaningful connections within the data. This includes patterns to identify, prioritize, and triage incidents faster.