How AIOps revolutionizes observability for TechOps teams
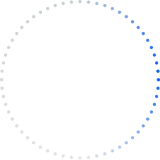
Managing over 1000 services and applications is daunting for any organization’s IT and tech operations team. With a diverse mix of on-premises legacy systems and modern cloud stacks, the sheer volume of activity can overwhelm even the most skilled ITOps teams. The task is made more difficult by the fact that observability is fragmented. On average, organizations depend on 21 systems that produce metrics, logs, traces, and alerts for various services.
This fragmentation leads to data overload, siloed operations, and inefficient or ineffective response to incidents. This post explores how applying AIOps (Artificial Intelligence for IT Operations) to observability can address these challenges, optimize service availability, reduce manual toil, increase efficiency, all while decreasing costs for SRE, DevOps, NOC, and ITOps teams.
The challenge of real-time observability overload
The real-time data generated by observability systems inundates IT teams, including NOCs, SREs, DevOps, and engineers responsible for service uptime. These teams are tasked with identifying actionable events and taking appropriate action.
However, contextualizing the observability data requires accessing and deciphering information from three fragmented subsets: topology systems, change systems, and historical operational data. Historical data is too often buried deep within organizations, resulting in delayed incident resolution and increased downtime.
AIOps as the solution
To effectively manage observability, organizations need a solution that can handle the diverse set of observability systems without requiring consolidation into a single system. BigPanda’s AIOps platform embraces this reality by ingesting data from all observability sources. Let’s explore how applying AIOps to observability enhances existing systems across three phases:
Phase 1: Compress and distill
AIOps enables the compression and distillation of events from metrics, logs, and traces. Rather than continuously monitoring these streams, individuals are notified when significant events or alerts occur. This shift from an eyes-on-glass approach to an alert-initiated mode is a game-changer for tech operations teams.
Reducing redundancies can significantly reduce the number of alerts and allows teams to focus on the most critical ones, saving time and reducing alert fatigue. Normalization standardizes data from different observability systems, creating a unified and consistent view of the information.
This single-pane-of-glass view provides better visibility and eliminates the need to navigate multiple tools. Filtering and suppression further manage diverse data sources and reduce information overload. By filtering out repetitive or irrelevant alerts, teams can concentrate on addressing more pressing issues. Historical data analysis informs filtering decisions, leveraging past incident data to determine the actionability of alerts and improve the efficiency of the response process.
Phase 2: Correlate and contextualize
Correlating and contextualizing data provides ITOps teams with a comprehensive understanding of events. Topological correlation examines alerts and identifies related events occurring in both time and topological proximity. This approach helps operators grasp the broader context of an event and understand its potential impact on the overall system. By investigating connected or nearby alerts within a network, teams gain a complete picture of what’s happening and can proactively prevent service impacts.
Topological enrichment furthers this understanding by providing all the topological relationships to downstream automated or human processing. This level of enrichment enables a more comprehensive analysis of the problem, identifies the affected elements, and determines their potential association with other elements. Operational enrichment brings critical organizational knowledge into the analysis, providing additional context and empowering teams to make informed decisions.
Phase 3: Predict, present, and automate
AIOps leverages historical data to predict and make recommendations based on prior incidents. By analyzing vast amounts of data, such as ticket information, run books, and post-mortems, AIOps can identify patterns and similarities to assist in incident diagnosis and resolution. This predictive capability enables ITOps teams to address potential issues before they impact service availability proactively. Clear and concise information presentation is crucial for efficient incident response. AIOps translates complex data from observability systems into easily readable and digestible formats. This includes providing insights into an incident’s impact on business services and applications, allowing teams to communicate effectively and respond promptly.
Auto-remediation is another significant advantage of AIOps. It identifies the root cause of an incident and relays the information to an automated system, such as Ansible or StackStorm, to autonomously fix the issue. This reduces manual toil, speeds up incident resolution, and delivers incident reports to humans for further analysis and learning.
Using AIOps for observability yields real-world results
Organizations that have embraced AIOps for observability have witnessed remarkable improvements in efficiency, service availability, and cost reduction. For example, InterContinental Hotels Group (IHG) achieved its highest-ever service availability by receiving actionable alerts in a centralized location, reducing response times and minimizing downtime. Bungie successfully compresses alerts by 99% using BigPanda Alert Intelligence, streamlining incident management and eliminating unnecessary noise. These real-world results demonstrate how AIOps streamlines incident management, reduces manual toil, optimizes resource allocation, and improves operational effectiveness.
Apply AIOps to observability for fully optimized service operations
By applying AIOps to observability, organizations can overcome data overload, reduce manual toil, increase efficiency, and decrease costs for SRE, DevOps, NOC, and ITOps teams. AIOps enables the compression and distillation of real-time data, provides contextual information for effective incident response, predicts and automates remediation, and streamlines incident management workflows.
The results speak for themselves, as organizations witness higher levels of service availability, reduced response times, and improved operational effectiveness. Learn how embracing AIOps in observability is the key to achieving optimized service operations in a world of ever-increasing complexity.