Does your cloud observability need AI-powered visibility?
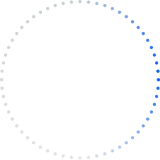
Maybe you’re still using monolithic applications, built and refined over many years. You understand that shifting to microservices or containerized architectures is a huge and daunting task. You’re probably grappling with the limitations of legacy systems—maybe they’re slow, tough to update, or can’t scale as you’d like. And you’re likely using more traditional IT monitoring tools or even some cloud observability tools. If some of these sound like you, you’re probably getting a patchy view of your infrastructure, leaving you with inefficiencies and hidden challenges. Isn’t it time for a change?
Clear cloud visibility is the answer. As you dive deeper into cloud architectures, ensuring these systems are efficient and reliable is essential. Solid cloud monitoring becomes crucial with the fast-paced changes across hybrid and multi-cloud tech, shifts in the competitive landscape, and ever-rising user expectations. Without proper observability and awareness, you risk affecting your revenue, reputation, and the trust your users place in your cloud setup.
Relying solely on cloud monitoring tools may disappoint in terms of real-time visibility, proactive incident management, managing your cloud infrastructure, and automation. However, that’s where AIOps comes in: AIOps helps to analyze the information from these tools and suggests automated actions.
So, we’ll look at how cloud monitoring and cloud observability work, the challenges of making observability actionable, and how AIOps provides cloud visibility with analytics and automation. With AIOps, you can easily see what’s happening in the cloud and get clear guidance on managing it to ensure peak application performance.
Read on to learn:
- Quick definition check: cloud monitoring vs cloud observability
- How cloud observability supports using containers
- What’s the difference between cloud versus on-prem monitoring?
- How AIOps simplifies hybrid, multi-cloud monitoring
- Five ways AIOps supports cloud visibility and data actionability
- AIOps brings visibility to your cloud infrastructure management
Quick definition check: cloud monitoring vs cloud observability
Before deciding whether you need enhanced cloud observability or an AI-powered solution, you need to understand standard cloud monitoring and how cloud observability builds upon it.
Cloud monitoring: Cloud monitoring collects, tracks, and analyzes metrics and logs from cloud-based resources and applications. It primarily focuses on ensuring systems are up and running, identifying when something goes wrong, and alerting relevant stakeholders. It answers the “what” – for instance, what is the current CPU usage? What’s the response time of a service?
Cloud observability: Cloud observability goes a step further than just monitoring. It provides a deeper understanding of the internal state of your systems by analyzing the external outputs. Observability lets you understand the “why” behind the metrics and logs, diving into the reasons for system behaviors. It encompasses logging, tracing, and metrics to provide a more holistic view of system health, performance, and potential bottlenecks.
In essence, while cloud monitoring gives you a surface-level view and alerts on system performance and issues, cloud observability provides a comprehensive understanding, allowing for more proactive troubleshooting and system optimization.
How cloud observability supports the use of containers
Cloud observability supports the use of containers through their granularity and scale, dynamic infrastructure, and service dependencies. With containers and microservices, applications often involve numerous moving parts distributed across various nodes. Microservices often communicate with each other, which introduces network dependencies.
Observability provides insights into these interactions, making spotting latency or failure points easier. Observability tools also help in monitoring, logging, and tracing activities across all these components. This granularity in observation is overkill for monolithic apps but is essential for modern distributed applications. However, this level of granularity can be a double-edged sword, providing so much data and creating so many alerts as to render them hard to address effectively.
What’s the difference between cloud versus on-prem monitoring?
The shift from monolithic to containerized apps has deep implications for approaching observability. Here are some of the essential differences between cloud versus on-prem monitoring.
- Infrastructure diversity: Cloud monitoring often has to account for a vast array of services offered by cloud providers, from serverless functions to managed databases. On-prem usually deals with a more fixed set of resources.
- Dynamic nature: Cloud environments are more dynamic. Resources can be provisioned or terminated on demand, scaling can be automated, and services might span multiple geographic regions. Monitoring needs to adapt to these rapid changes.
- Access and control: With on-prem systems, organizations have physical control over their hardware and often deeper insights into the system. In the cloud, some underlying details might be abstracted away by the cloud provider, requiring different monitoring approaches.
- Cost implications: Cloud services often follow a consumption, pay-as-you-go model. Monitoring can help in identifying cost hotspots, ensuring efficient resource utilization. In on-prem environments, the primary costs are often sunk costs related to purchasing and maintaining the hardware.
- Integration with native tools: Cloud providers offer their own monitoring solutions (e.g., AWS CloudWatch). Leveraging these tools and integrating them with third-party solutions might introduce complexities not present in on-prem setups.
As systems become more distributed and dynamic, especially in cloud environments, organizations need cohesive, end-to-end visibility into applications and services spanning hybrid IT stacks. For this reason, companies move beyond cloud observability to cloud visibility and AIOps for insight, intelligence, and automation.
How AIOps simplifies hybrid, multi-cloud monitoring
AIOps brings intelligent automation to IT operations, leveraging advanced algorithms and machine learning to improve the visibility and actionability of data. Especially in the context of cloud infrastructure, AIOps can play a pivotal role in simplifying monitoring and ensuring operational excellence. Here are the key capabilities AIOps enables:
- Scalability and dynamism: Cloud environments can be highly dynamic, with resources spinning up or down based on demand. AIOps can adapt to these fluctuations, ensuring monitoring remains consistent and relevant.
- Integration across services: Cloud platforms consist of various services, from compute instances to managed databases. AIOps can aggregate data across these services, offering a holistic view of the environment.
- Unified monitoring across hybrid environments: Many organizations operate in hybrid environments, leveraging both on-prem and cloud resources. AIOps can provide a unified monitoring solution, bridging the gap between these two realms.
- Cost efficiency: By predicting issues, reducing noise, and speeding up troubleshooting, AIOps can contribute to more efficient resource utilization in the cloud, potentially leading to cost savings.
- Continuous learning: The cloud is ever-evolving, with new services and features introduced regularly. AIOps platforms can learn continuously, adapting to new data sources and refining their analyses over time.
Five ways AIOps supports cloud visibility and data actionability
AIOps enhances cloud monitoring by intelligently identifying and distinguishing between critical issues and minor anomalies, ensuring that IT teams focus on the most pressing matters. By supporting visibility and actionability, AIOps can proactively address potential problems with your cloud infrastructure management. Here are the five ways that AIOps makes this possible.
- Ingesting all types of cloud data: AIOps platforms use advanced algorithms and machine learning to analyze the vast amounts of data generated by hybrid cloud monitoring environments. By identifying patterns and establishing baselines, AIOps can identify these systems and detect deviations or anomalies, often before they lead to significant issues or outages.
- Event correlation and noise reduction: Traditional monitoring systems can produce a lot of noise in the form of redundant or non-critical alerts. AIOps can group related alerts and events, correlating them to a single root cause, reducing noise, and ensuring that IT teams focus on real issues.
- Root cause analysis (RCA): Instead of just identifying a problem, AIOps can analyze the relationships between systems and changes made across your CI/CD pipeline to automate root cause analysis and suggest probable root causes to drastically speed up the troubleshooting process.
- Hybrid cloud visibility: AIOps facilitates detecting, investigating, and resolving incidents in hybrid IT infrastructures. Using real-time topology data from modern and legacy sources, AIOps creates a continuously updated full-stack topology model. This aids in contextual enrichment, correlation, and impact mapping in dynamic and cloud architectures, surpassing traditional CMDB limitations.
Rationalizing tools vs. adding complexity: Instead of having multiple monitoring solutions, each focusing on specific aspects or generating alerts, AIOps integrates data from various sources for a unified view. By reducing noise, correlating events, and streamlining analyses, AIOps tools simplify the monitoring landscape rather than adding another layer of complexity.
This Sankey diagram demonstrates how BigPanda AIOps reduces alert noise to give teams better visibility and control.
Ultimately, AIOps support for cloud visibility integrates both actionability and data awareness. This ensures the vast amounts of data generated in the cloud infrastructure are informative and can trigger automated corrective measures, streamlining operations and minimizing manual interventions.
Gain visibility to your cloud infrastructure management with AIOps
At BigPanda, we’ve crafted an AIOps solution that harnesses the power of advanced algorithms and machine learning, so you can easily sift through vast amounts of cloud data to spotlight vital issues for swift detection and improved MTTR.
BigPanda provides awareness across your hybrid IT environments, spanning legacy, homegrown, on-prem, and cloud infrastructures. Our platform offers cross-domain correlation, enrichment, and AI/ML to identify impact mapping within dynamic architectures. See for yourself in our personalized demo how BigPanda can give you a complete understanding of your cloud stack for better efficiency, actionability, and availability.