Use AIOps and anomaly detection to speed incident resolution
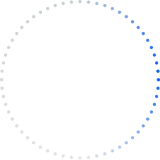
Quickly finding and resolving monitoring anomalies can make all the difference between service issues — and service excellence. But it’s far from easy, whether you’re trying to sift through countless alerts, understand the context behind anomalies, or swiftly pinpoint their root causes.
If you’re an ITOps practitioner or enterprise architect looking to fine-tune your anomaly detection and resolution skills, you’ve come to the right place. In this post, we’ll explore essential topics such as challenges in anomaly detection, using observability tools for detection, and how AIOps facilitates faster and more efficient resolution.
What is anomaly detection in monitoring and observability?
Anomaly detection refers to the process of automatically identifying patterns or behaviors within IT systems that deviate from the norm. Anomaly detection can be found across data sources, including logs, metrics, events, time series data, user behavior, external data, and configuration data.
How observability tools detect anomalies
Observability tools give insight into the health of services by answering essential questions about what’s slow, what’s broken, and what needs attention to enhance performance.
Given their domain knowledge and data sets, monitoring and observability tools are highly suitable at quickly detecting and understanding deviations and anomalies in performance.
Anomaly detection shouldn’t be confused with predictive analysis. While anomaly detection focuses on identifying abnormal behavior as it occurs, predictive analysis instead takes historical data and uses it to forecast future events and trends.
Challenges with detecting anomalies in IT operations
Anomaly detection is essential for catching issues before they escalate, but it’s not without its challenges. Here are some of the most common hurdles teams face when improving their anomaly detection.
- Data quality: Garbage in, garbage out. Low-quality data with inconsistencies, inaccuracies, and missing values can mislead algorithms and lead to false positives or negatives. This means you must clean and structure data to ensure effective analysis.
- Noise and false alarms: Complex IT environments generate a constant stream of events, some normal and some abnormal. Distinguishing true indicators of trouble from the noise is tricky. Tuning algorithms to avoid sensitive thresholds that trigger too many false alerts is essential.
- Limited training data: Anomaly detection algorithms learn by example. Insufficient or poorly labeled training data can hinder their ability to recognize subtle patterns and differentiate between normal and abnormal behavior. Overcome this by expanding and enriching your training data sets.
- Domain expertise: Interpreting anomalies and determining their severity requires context and expert knowledge. Even sophisticated algorithms need human input to understand the nuances of specific systems and situations. Collaboration between data scientists and IT operations teams is vital.
- Variable baselines: IT systems have inherent seasonality, trends, and unexpected fluctuations. This makes defining a clear “normal” state challenging, often leading to false positives or missed anomalies.
To significantly improve your anomaly detection, you must overcome these obstacles. Doing so will require combining high-quality data, sophisticated algorithms, human expertise, and a willingness to embrace new approaches.
What is anomaly detection with machine learning?
Many ITOps teams use AI/ML within their observability tools to examine data to identify abnormal behavior. This behavior includes trends and patterns that traditional threshold-based alerting might miss when a metric deviates from its expected behavior.
By leveraging machine learning, anomaly detection can instantly identify possible abnormal activity, giving engineers and developers a heads-up about potential service issues.
AIOps, anomaly detection, and observability
Observability and anomaly detection are two sides of the same coin in AIOps. They are not standalone solutions but complementary tools.
When integrated effectively, using purpose-built AIOps platforms together with your observability solutions can significantly reduce service downtime and create a more proactive IT environment. Here’s how:
- Consistency and accuracy: AIOps enables teams to trust their alerts, which may come from anomaly detecting, so they can focus on genuine issues rather than false alarms.
- Alert fatigue: Too many alerts can overwhelm IT teams, leading to alert fatigue and missed critical events. AIOps lets you streamline and prioritize alerts.
- Understanding root cause: Identifying the root cause of an anomaly can be difficult, requiring further investigation and correlation with other data sources. AIOps uses AI/ML to correlate your observability data with other sources and suggest incident root cause in real-time.
Use AIOps for anomaly detection and incident resolution
Standard monitoring tools primarily focus on tracking predefined metrics and logs to maintain system health. However, by using AI to learn from data patterns, enabling it to identify unexpected issues that standard monitoring might miss, you can go from simple anomaly detection to speedier incident resolution with AIOps.
AIOps enhances anomaly resolution by expediting the journey from alert recognition to automated resolution processes, resulting in notable downtime reductions and improved operational efficiency.
This AI-driven approach can adapt to new threats and changes in the IT environment, providing a more dynamic and proactive defense against issues. In a previous webinar on anomaly detection and observability, put it this way:
“Configure your system so that anything that it spits out into your event management pipeline gets correlated with the things that caused it […] so your team can quickly take action on it.”
Through its real-time provision of contextual insights, automated root cause analysis, and the delivery of actionable guidance, AIOps empowers IT teams to swiftly address issues and mitigate their impact on business operations.
Tools for anomaly detection: Log vs metric analysis tools
AIOps can seamlessly integrate with Log and Metric Analysis Tools, using the power of log data for detailed context and metrics for real-time performance monitoring. There are two main types of anomaly detection tools: log analysis tools and metric analysis tools.
- Log analysis tools help you spot unusual event patterns within the vast sea of data stored in log files. Log analysis tools primarily analyze textual log files generated by IT systems, applications, and devices. Log analysis tools provide detailed insights into events, errors, and activities in textual form, allowing for a deep understanding of the context of anomalies.
- Metric analysis tools focus on identifying abnormal behavior in time-series metrics, considering factors like the time of day and changing application patterns. Metric analysis tools primarily analyze numerical performance metrics and time-series data, such as CPU usage, network traffic, and response times. These tools also focus on numeric patterns and trends, making them more suitable for detecting performance-related anomalies.
This dual approach ensures comprehensive anomaly detection so your IT teams can swiftly identify and resolve issues from log discrepancies or irregular metric patterns.
BigPanda AIOps and anomaly detection
BigPanda provides improved anomaly detection by integrating AI and machine learning into IT systems management. It analyzes vast amounts of operational data to identify unusual patterns or discrepancies that could signify problems.
Because BigPanda is not an observability tool, it has a distinct view into your observability and monitoring stack and can uniquely deliver more from your observability investments. BigPanda can help you evaluate, tune, and improve your data sources. We can also:
- Correlate alerts from different sources and group related anomalies to avoid alert fatigue so IT teams can focus on critical issues.
- Provide AI-powered root cause analysis to pinpoint the source of an anomaly
- Leverage insights into observability gaps and redundancies to make your anomaly detection more impactful
Interested in learning more about BigPanda, how you can get more from your observability and monitoring investments, and make observability more actionable? Request your personalized demo.